Research
Deep learning model can predict the orientation of nanoparticles
November 24, 2020
New imaging platform offers valuable data for development of ‘smart’ drug delivery and next-generation therapeutic systems
Nanoparticles are almost unfathomably small, beyond the sight of ordinary microscopes. But the ability to track their movements is one of the keys to improving medical diagnosis and delivering targeted, effective patient care.
Now, with a new deep learning model, researchers at Northwestern University can determine the 3D orientation of a nanoparticle. The model can predict a nanoparticle’s angle of rotation at any given point, including its interaction with a human cell – quickly and accurately.
“We wanted to combine advances in imaging techniques, nanoparticle design, and computational analysis into a particle-tracking platform,” said Teri W. Odom, who led the research. “The imaging platform can provide predictive nanoparticle orientation information that can be directly applied to how a nanoparticle carrying medication can target a specific cell – and potentially even the movement of molecules within those cells.”
The study was recently published (Nov. 9) in the journal ACS Central Science.
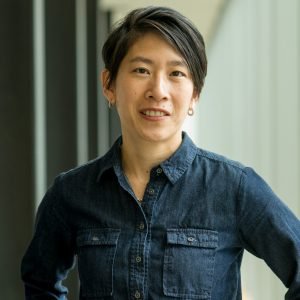
Odom is the chair of Northwestern’s chemistry department and the Charles E. and Emma H. Morrison Professor of Chemistry in the Weinberg College of Arts and Sciences. She is also an affiliated faculty member of Northwestern’s International Institute for Nanotechnology. Jingtian Hu, a postdoctoral fellow in Odom’s laboratory, is the paper’s first author.
Nanomedicine has shown incredible promise for a wide range of medical applications, from early diagnosis of diseases to targeted drug delivery without harming cellular environments. For example, spherical nucleic acids (SNAs) are a globular nanoparticle form of DNA that can enter and stimulate immune cells against diseases like cancer.
Optical microscopy is crucial for development of these treatments. In particular, the rotational motions of a nanoparticle provide critical information on how the particle targets specific cells. The difference between how a nanoparticle interacts with a healthy cell compared to a cancer cell is essential to understanding the nanoparticle’s effectiveness as a therapeutic agent.
However, tracking nanoparticle rotations is challenging – not only because of the comparatively limited resolution of optical microscopes, but also the lack of analysis methods that can reliably extract the three-dimensional orientation of a nanoparticle from a two-dimensional microscopy image. A scientist can do this by manually comparing a new image to a series of other images where the orientation is already known, but the process is time-consuming, even more so if the nanoparticle was out-of-plane with the camera in the new image.
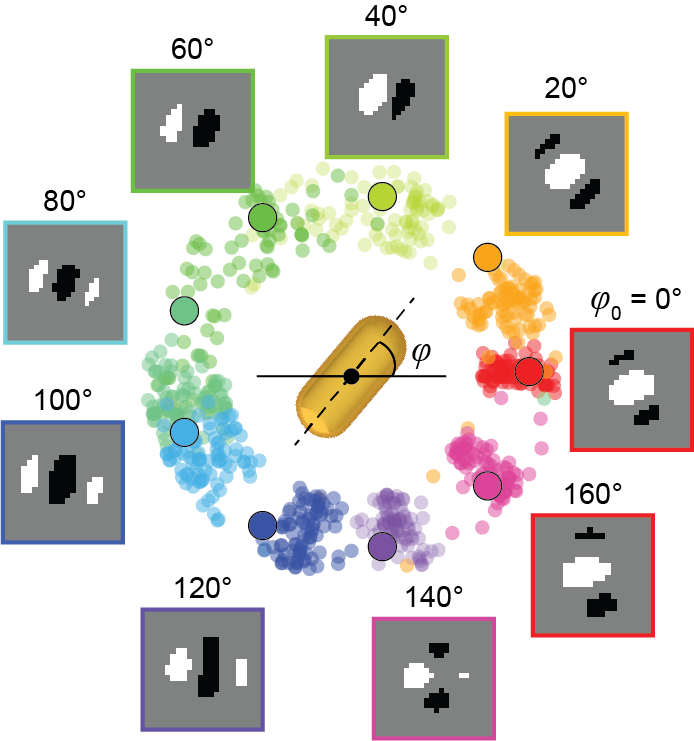
Odom’s team saw an opportunity to create a model that could perform this analysis, using deep learning – a technique that teaches computers to learn by example. They began by constructing a large dataset of images of non-symmetrical gold nanostars observed with differential interference contrast (DIC) microscopy. Then, they labeled the orientation of the nanoparticle in each image and trained the model to interpret the images.
Using what it “learned” from that dataset, the resulting model can look at a new DIC image and predict the orientation of a nanoparticle – much faster than a scientist could, with a degree of accuracy that is limited only by the capabilities of the imaging instrument.
Going forward, Odom’s team plans to continue training the model to interpret images and improve its ability to generate data about 3D rotational dynamics. Having more data about the behavior of nanoparticles used to carry medication or signal the presence of a disease in a cell will inform the design of those particles, making them more effective in future applications.
“We believe this model could also be used in the study of fundamental biological problems,” said Odom. “Every human cell contains many different types of molecules, and there’s a need to better understand the movement of those molecules and their assemblies. Our deep-learning model for optical probes can a provide a window into how cells move things around and what that might mean for human health.”
The paper, “Single-Nanoparticle Orientation Sensing by Deep Learning,” was supported by the National Science Foundation (award CMMI-1848613) and National Institutes of Health (grant 5 R01 GM131421-02), as well as the EPIC and NUFAB facility of Northwestern University’s NUANCE Center; the MRSEC program (NSF DMR-1720139) at the Materials Research Center; the International Institute for Nanotechnology; and the Keck Foundation.